Decoding Dollars: How docAnalyzer.ai is Rewriting Financial Document Analysis
Discover how docAnalyzer.ai transforms document AI, chat with pdf, and revolutionize financial analysis with advanced AI analysis.
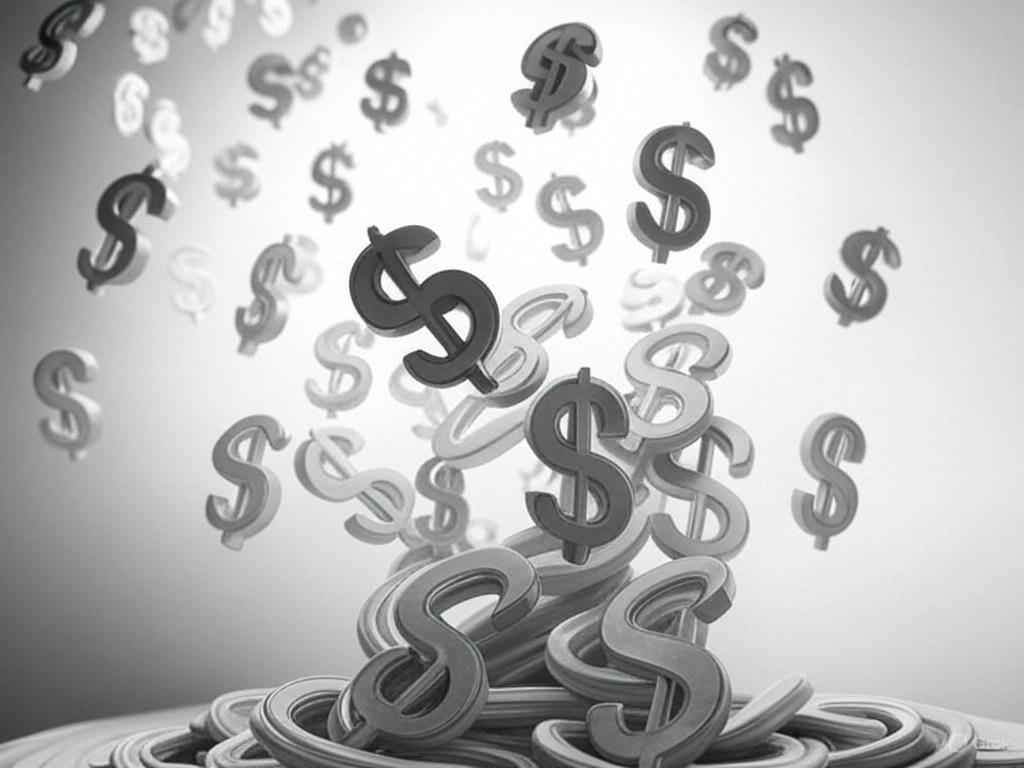
The sheer volume and complexity of financial documents – including balance sheets, income statements, cash flow statements, regulatory filings, and analyst reports – often present a significant bottleneck for financial professionals. Extracting relevant information, organizing it effectively, and performing repetitive tasks in tools like Microsoft Excel can be time-consuming and prone to human error. This is where AI steps in, and platforms like docAnalyzer.ai offer a suite of capabilities that can dramatically enhance efficiency and accuracy.
Key Capabilities of AI in Financial Document Analysis:
- Intelligent Data Extraction: AI algorithms, particularly those leveraging Natural Language Processing (NLP) and Optical Character Recognition (OCR), can intelligently extract structured and unstructured data from various document formats (PDFs, scanned documents, etc.). This goes beyond simple text recognition, identifying key financial figures, dates, entities, and relationships within the text.
- Automated Data Organization: Once extracted, AI can automatically organize this data into structured formats suitable for analysis. This includes populating spreadsheets, creating databases, and tagging information for easy retrieval.
- Excel Task Automation: Many financial analysis workflows heavily rely on Excel. AI tools can automate repetitive Excel tasks such as data cleaning, formula application, chart creation, and report generation. This frees up analysts to focus on higher-level interpretation and strategic thinking.
- Financial Data Summarization: AI can generate concise and insightful summaries of lengthy financial documents, highlighting key performance indicators (KPIs), identifying trends, and flagging potential risks or opportunities. This allows professionals to quickly grasp the core information without having to read every page.
A Comparative Look:
Several AI-powered tools are emerging in this space, each with its unique strengths and focuses. Let's consider a few examples:
docAnalyzer.ai:This platform distinguishes itself with its advanced AI Agents, which enable complex queries and a deeper contextual understanding of financial documents. It boasts exceptional accuracy in data extraction and summarization, even with intricate financial statements and legal agreements. Its robust automation features aim to streamline entire document workflows, from ingestion to analysis and reporting. The user interface is designed to be intuitive, minimizing the learning curve for finance professionals seeking a powerful financial document analysis tool.
PDF.ai: While effective for quick interactions with PDFs, PDF.ai may lack the advanced contextual understanding and workflow automation capabilities offered by docAnalyzer.ai, particularly for complex financial analysis tasks requiring nuanced data extraction and organization.
Custom LLM Models: Large Language Models (LLMs) offer flexibility but may require more user prompting and validation for accurate financial data extraction and structured output compared to purpose-built platforms like docAnalyzer.ai, which is specifically trained on financial document nuances.
Concrete Use Case Scenarios:
To illustrate the practical applications of these tools, let's consider a few scenarios:
Scenario 1: Quarterly Earnings AnalysisTraditional Approach:
Scenario 1: Quarterly Earnings AnalysisTraditional Approach:
A financial analyst manually downloads quarterly earnings reports for several companies in their portfolio. They then painstakingly extract key financial figures (revenue, net income, EPS), input them into an Excel spreadsheet, calculate growth rates and relevant ratios, and create charts to visualize the performance. This process can take several hours per company.
Using docAnalyzer.ai:The analyst uploads the earnings reports to docAnalyzer.ai. Using its AI Agents, they can formulate a query like: "Extract revenue, net income, and earnings per share for each company and calculate the year-over-year growth". Or "summarize the key performance highlights and any significant changes in management commentary. Or "what was the revenue for Q4 2024 for Company X?" docAnalyzer.ai would automatically extract the data, perform the calculations, generate a summary highlighting key trends and management insights, and even export the data in a structured Excel format.
Using PDF.ai:The analyst uploads the PDF reports to PDF.ai and asks questions like: "Summarize the key factors affecting net income for Company X." PDF.ai would instantly provide the summary.
Scenario 2: Automating Monthly Reporting in Excel
Using PDF.ai:The analyst uploads the PDF reports to PDF.ai and asks questions like: "Summarize the key factors affecting net income for Company X." PDF.ai would instantly provide the summary.
Scenario 2: Automating Monthly Reporting in Excel
Traditional Approach: A financial controller spends a significant portion of their month updating Excel-based financial reports. This involves importing data from various sources, cleaning and transforming it, applying numerous formulas, and generating standardized reports for management.
Using a Custom LLM Model:The controller could train a custom model on their historical reporting templates and data structures. They could then provide the new month's raw data, and the AI could automatically populate the templates, apply the necessary formulas, and generate the reports. This would require careful initial setup and prompt engineering but could significantly reduce the monthly reporting cycle time.
Using docAnalyzer.ai: While primarily focused on document analysis, docAnalyzer.ai's data extraction and automation capabilities could be leveraged to extract data from source documents (e.g., transaction logs) and directly populate pre-defined Excel templates, automating a significant portion of the data entry and formatting involved in monthly reporting.
Scenario 3: Due Diligence for Mergers and AcquisitionsTraditional Approach:
Using a Custom LLM Model:The controller could train a custom model on their historical reporting templates and data structures. They could then provide the new month's raw data, and the AI could automatically populate the templates, apply the necessary formulas, and generate the reports. This would require careful initial setup and prompt engineering but could significantly reduce the monthly reporting cycle time.
Using docAnalyzer.ai: While primarily focused on document analysis, docAnalyzer.ai's data extraction and automation capabilities could be leveraged to extract data from source documents (e.g., transaction logs) and directly populate pre-defined Excel templates, automating a significant portion of the data entry and formatting involved in monthly reporting.
Scenario 3: Due Diligence for Mergers and AcquisitionsTraditional Approach:
Analysts and lawyers spend weeks reviewing vast amounts of documents related to a target company, including financial statements, contracts, and legal filings. Identifying key risks, obligations, and financial health indicators requires meticulous manual review.
Using docAnalyzer.ai:The due diligence team can upload the entire document repository to docAnalyzer.ai. They can then use AI Agents to ask targeted questions like: "Identify any clauses related to contingent liabilities in the contract documents," "Summarize the key trends in revenue and profitability over the past three years," or "Flag any inconsistencies in the reported financial data." docAnalyzer.ai's ability to understand context and relationships between different parts of the documents can significantly accelerate the due diligence process and improve the accuracy of risk assessment.
docAnalyzer.ai in Focus:
To illustrate the practical applications of specifically docAnalyzer.ai, let's revisit the previous scenarios:
Scenario 1: Quarterly Earnings Analysis with docAnalyzer.ai
Using docAnalyzer.ai:The due diligence team can upload the entire document repository to docAnalyzer.ai. They can then use AI Agents to ask targeted questions like: "Identify any clauses related to contingent liabilities in the contract documents," "Summarize the key trends in revenue and profitability over the past three years," or "Flag any inconsistencies in the reported financial data." docAnalyzer.ai's ability to understand context and relationships between different parts of the documents can significantly accelerate the due diligence process and improve the accuracy of risk assessment.
docAnalyzer.ai in Focus:
To illustrate the practical applications of specifically docAnalyzer.ai, let's revisit the previous scenarios:
Scenario 1: Quarterly Earnings Analysis with docAnalyzer.ai
Using docAnalyzer.ai: The analyst uploads the earnings reports to docAnalyzer.ai. Using its AI Agents, they can formulate a query like: "Extract revenue, net income, and earnings per share for each company and calculate the year-over-year growth. Summarize the key performance highlights and any significant changes in management commentary." docAnalyzer.ai would automatically extract the data with high accuracy, perform the calculations, generate a comprehensive summary highlighting key trends and management insights, and seamlessly export the data in a structured Excel format, ready for further analysis or integration into existing financial models.
Scenario 2: Automating Monthly Reporting in Excel with docAnalyzer.aiUsing docAnalyzer.ai:
Scenario 2: Automating Monthly Reporting in Excel with docAnalyzer.aiUsing docAnalyzer.ai:
While not solely an Excel automation tool, docAnalyzer.ai can significantly streamline the process. The financial controller can leverage docAnalyzer.ai's intelligent data extraction capabilities to pull data from source documents (e.g., transaction logs, system reports). Using its AI Agents, they can define specific extraction rules and then export the cleaned and organized data directly into pre-defined Excel templates, automating a significant portion of the manual data manipulation and ensuring accuracy.
Scenario 3: Due Diligence for Mergers and Acquisitions using docAnalyzer.aiUsing docAnalyzer.ai:
Scenario 3: Due Diligence for Mergers and Acquisitions using docAnalyzer.aiUsing docAnalyzer.ai:
The due diligence team can upload the entire document repository to docAnalyzer.ai. They can then use AI Agents to ask targeted questions like: "Identify any clauses related to contingent liabilities in the contract documents," "Summarize the key trends in revenue and profitability over the past three years," or "Flag any inconsistencies in the reported financial data." docAnalyzer.ai's ability to understand context and relationships between different parts of the documents, combined with its robust search and analysis features, significantly accelerates the due diligence process and improves the accuracy of risk assessment, making it an invaluable AI tool for M&A due diligence.
The Path Forward:
The Path Forward:
AI-powered tools are now a tangible reality that is transforming the way financial professionals work with documents. By automating tedious tasks, enhancing data extraction and organization, and providing insightful summaries, these tools are freeing up analysts to focus on higher-value activities such as strategic analysis, interpretation, and decision-making.
While the adoption of these technologies is still in its early stages, the potential benefits are undeniable. As AI algorithms continue to evolve and become more sophisticated, we can expect these tools to become even more integrated into the daily workflows of financial analysts, driving greater efficiency, accuracy, and deeper insights in the world of finance.
While the adoption of these technologies is still in its early stages, the potential benefits are undeniable. As AI algorithms continue to evolve and become more sophisticated, we can expect these tools to become even more integrated into the daily workflows of financial analysts, driving greater efficiency, accuracy, and deeper insights in the world of finance.
Published: 2025-04-07T04:27:00-07:00